Analyzing the Impact of Algorithmic Bias on the Financial Industry
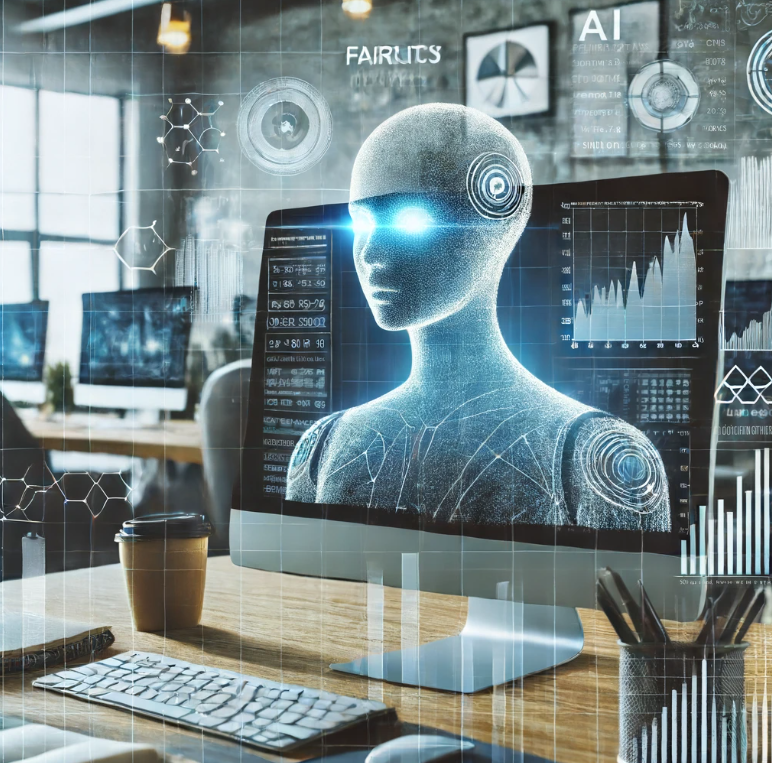
As artificial intelligence (AI) continues to integrate into various industries, the risk of algorithmic bias becomes increasingly apparent. The attached article, "Designing AI for All: A Primer on Bias in Artificial Intelligence Systems," highlights numerous instances where AI systems have exhibited significant biases, leading to discriminatory outcomes. This analysis focuses on the financial industry, examining the potential impact of algorithmic bias on organizations and careers within this sector.
Industry Impact
Algorithmic bias in the financial industry can have far-reaching consequences, affecting credit scoring, loan approvals, and investment decisions. For instance, biased algorithms can result in discriminatory lending practices, where minority groups may be unfairly denied credit or offered less favorable terms compared to their white counterparts. Such biases can exacerbate existing financial inequalities, making it harder for affected individuals to access essential financial services.
Algorithmic bias in the financial industry can have far-reaching consequences, affecting credit scoring, loan approvals, and investment decisions. For instance, biased algorithms can result in discriminatory lending practices, where minority groups may be unfairly denied credit or offered less favorable terms compared to their white counterparts. Such biases can exacerbate existing financial inequalities, making it harder for affected individuals to access essential financial services.
Organizational Challenges
Organizations within the financial industry must address algorithmic bias proactively to maintain trust and ensure compliance with regulatory standards. Regulatory bodies are increasingly scrutinizing AI systems for fairness and transparency, and failure to comply can lead to hefty fines and reputational damage. For instance, the European Union's General Data Protection Regulation (GDPR) and the upcoming AI Act emphasize the need for transparency and non-discrimination in automated decision-making processes.
To mitigate bias, financial institutions must implement rigorous testing and auditing of their AI systems. This includes regular assessments to identify and rectify biases in training data, model selection, and deployment strategies. Institutions should also invest in diverse teams of data scientists and AI researchers to bring varied perspectives and reduce the risk of unintentional bias.
Career Implications
The rise of AI and the need to address algorithmic bias present both opportunities and challenges for careers in the financial industry. On one hand, there is an increasing demand for professionals skilled in AI ethics, fairness, and transparency. Data scientists, AI engineers, and compliance officers with expertise in these areas will be highly sought after as organizations strive to develop and maintain fair AI systems.
On the other hand, the presence of algorithmic bias may lead to job displacement and require significant upskilling for current employees. As AI systems take on more decision-making roles, financial professionals must adapt by acquiring new skills in AI and data analytics. Continuous learning and
professional development will be crucial to stay relevant in a rapidly evolving industry.
Conclusion
Algorithmic bias presents a significant challenge to the financial industry, with the potential to perpetuate inequalities and undermine trust in AI systems. However, by proactively addressing these biases through
rigorous testing, diverse team composition, and regulatory compliance, financial institutions can harness the benefits of AI while ensuring fairness and transparency. For professionals in the industry, this shift underscores the importance of continuous learning and ethical awareness, paving the way for a
more equitable and trustworthy financial ecosystem.